Time Series Data Sets Download
Time Series Regression of Airline Passenger Data
- Time Series Data Sets
- Financial Time Series Data Sets
- Time Series Data Sets Download Free
- Download Time Series Data
- Time Series Data Sets Download Free
- Sample Time Series Data Set
South Africa interactive database of downloadable datasets Country profiles. A very long time series: GDP growth in Sweden (1720-2000). Time Series data sets (2013) A new compilation of data sets to use for investigating time series data. File Size: 5 kb. File Type: csv. Download File. This example shows how to analyze time series data using Statistics and Machine Learning Toolbox™ features. Toggle Main Navigation. Time Series Regression of Airline Passenger Data. Unlike in the usual regression model when we would like the residual series r(t) to be a set of independent values. The Time Series Data Library is a collection of about 800 time series that I have maintained since about 1992, and hosted on my personal website. It includes data from a lot of time series textbooks, as well as many other series that I’ve either collected for student projects or helpful people have sent to me. Business and Industry. Census.gov › Business & Industry › Time Series / Trend Charts › Download Data Sets Main. DOWNLOAD DATA SETS. Feb 15, 2019 - You must create a free account on the site to download data. Use Sign Up to. Free time-series data sets include: historical workstation sales,.
8 Useful Databases to Dig for Data (and 100 more) Ai Ching CEO| Piktochart. Free time-series and micro-data to download (tags: economics, links) Official Google Blog. “uspop2002″ data set (tags: music, similarity, machinelearning).
This example shows how to analyze time series data using Statistics and Machine Learning Toolbox™ features.
Air Passenger Data
First we create an array of monthly counts of airline passengers, measured in thousands, for the period January 1949 through December 1960.
Create Time Series Object
When we create a time series object, we can keep the time information along with the data values. We have monthly data, so we create an array of dates and use it along with the Y data to create the time series object.
Examine Trend and Seasonality
This series seems to have a strong seasonal component, with a trend that may be linear or quadratic. Furthermore, the magnitude of the seasonal variation increases as the general level increases. Perhaps a log transformation would make the seasonal variation be more constant. First we'll change the axis scale.
It appears that it would be easier to model the seasonal component on the log scale. We'll create a new time series with a log transformation.
Now let's plot the yearly averages, with monthly deviations superimposed. We want to see if the month-to-month variation within years appears constant. For these manipulations treating the data as a matrix in a month-by-year format, it's more convenient to operate on the original data matrix.
Now let's reverse the years and months, and try to see if the year-to-year trend is constant for each month.
Model Trend and Seasonality
Let's attempt to model this series as a linear trend plus a seasonal component.
Time Series Data Sets
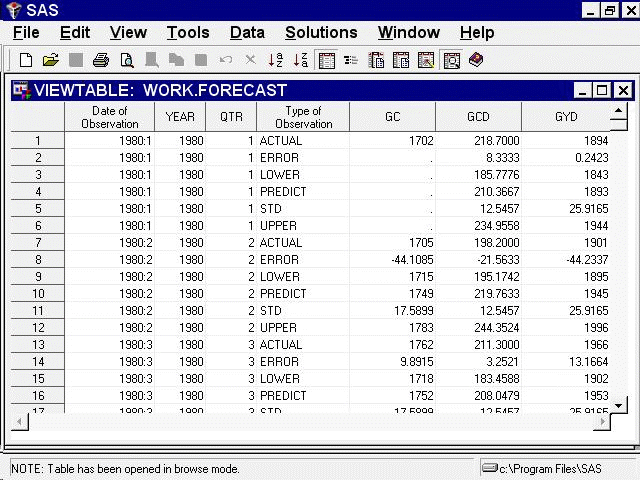
Based on this graph, the fit appears to be good. The differences between the actual data and the fitted values may well be small enough for our purposes.
But let's try to investigate this some more. We would like the residuals to look independent. If there is autocorrelation (correlation between adjacent residuals), then there may be an opportunity to model that and make our fit better. Let's create a time series from the residuals and plot it.
Financial Time Series Data Sets
The residuals do not look independent. In fact, the correlation between adjacent residuals looks quite strong. We can test this formally using a Durbin-Watson test.
Time Series Data Sets Download Free
A low p-value for the Durbin-Watson statistic is an indication that the residuals are correlated across time. A typical cutoff for hypothesis tests is to decide that p<0.05 is significant. Here the very small p-value gives strong evidence that the residuals are correlated.
We can attempt to change the model to remove the autocorrelation. The general shape of the curve is high in the middle and low at the ends. This suggests that we should allow for a quadratic trend term. However, it also appears that autocorrelation will remain after we add this term. Let's try it.
Adding the squared term did remove the pronounced curvature in the original residual plot, but both the plot and the new Durbin-Watson test show that there is still significant correlation in the residuals.
Autocorrelation like this could be the result of other causes that are not captured in our X variable. Perhaps we could collect other data that would help us improve our model and reduce the correlation. In the absence of other data, we might simply add another parameter to the model to represent the autocorrelation. Let's do that, removing the squared term, and using an autoregressive model for the error.
In an autoregressive process, we have two stages:
Unlike in the usual regression model when we would like the residual series r(t)
to be a set of independent values, this model allows the residuals to follow an autoregressive model with its own error term u(t)
that consists of independent values.
Download Time Series Data
To create this model, we want to write an anonymous function f
to compute fitted values Yfit
, so that Y-Yfit
gives the u values:
Time Series Data Sets Download Free
In this anonymous function we combine [rho; b]
into a single parameter vector c
. The resulting residuals look much closer to an uncorrelated series.
Summary
This example provides an illustration of how to use the MATLAB® timeseries object along with features from the Statistics and Machine Learning Toolbox. It is simple to use the ts.data
notation to extract the data and supply it as input to any function. The controlchart
function also accepts time series objects directly.
Sample Time Series Data Set
More elaborate analyses are possible by using features specifically designed for time series, such as those in Econometrics Toolbox™ and System Identification Toolbox™.